Comprehensive Evaluation of Matrix Factorization Models for Collaborative Filtering Recommender Systems
Autor:
Bobadilla, Jesús
; Dueñas-Lerín, Jorge
; Ortega, Fernando
; Gutiérrez, Abraham
Fecha:
06/2024Palabra clave:
Revista / editorial:
International Journal of Interactive Multimedia and Artificial IntelligenceCitación:
Jesús Bobadilla, Jorge Dueñas-Lerín, Fernando Ortega, Abraham Gutiérrez (2024). "Comprehensive Evaluation of Matrix Factorization Models for Collaborative Filtering Recommender Systems", International Journal of Interactive Multimedia and Artificial Intelligence, vol. 8, issue Regular Issue, no. 6, pp. 15-23. https://doi.org/10.9781/ijimai.2023.04.008Tipo de Ítem:
article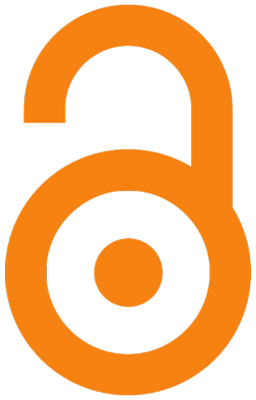
Resumen:
Matrix factorization models are the core of current commercial collaborative filtering Recommender Systems. This paper tested six representative matrix factorization models, using four collaborative filtering datasets. Experiments have tested a variety of accuracy and beyond accuracy quality measures, including prediction, recommendation of ordered and unordered lists, novelty, and diversity. Results show each convenient matrix factorization model attending to their simplicity, the required prediction quality, the necessary recommendation quality, the desired recommendation novelty and diversity, the need to explain recommendations, the adequacy of assigning semantic interpretations to hidden factors, the advisability of recommending to groups of users, and the need to obtain reliability values. To ensure the reproducibility of the experiments, an open framework has been used, and the implementation code is provided.
Ficheros en el ítem
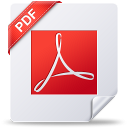
Nombre: Comprehensive Evaluation of Matrix Factorization Models for Collaborative Filtering Recommender Systems.pdf
Tamaño: 4.844Mb
Formato: application/pdf
8 readers on Mendeley
Este ítem aparece en la(s) siguiente(s) colección(es)
Estadísticas de uso
Año |
2012 |
2013 |
2014 |
2015 |
2016 |
2017 |
2018 |
2019 |
2020 |
2021 |
2022 |
2023 |
2024 |
2025 |
Vistas |
0 |
0 |
0 |
0 |
0 |
0 |
0 |
0 |
0 |
0 |
0 |
168 |
349 |
30 |
547 |
Descargas |
0 |
0 |
0 |
0 |
0 |
0 |
0 |
0 |
0 |
0 |
0 |
190 |
166 |
50 |
406 |
Ítems relacionados
Mostrando ítems relacionados por Título, autor o materia.
-
Classification-based Deep Neural Network Architecture for Collaborative Filtering Recommender Systems
Bobadilla, Jesús; Ortega, Fernando; Gutiérrez, Abraham; Alonso, Santiago (International Journal of Interactive Multimedia and Artificial Intelligence (IJIMAI), 03/2020)This paper proposes a scalable and original classification-based deep neural architecture. Its collaborative filtering approach can be generalized to most of the existing recommender systems, since it just operates on the ... -
A Collaborative Filtering Probabilistic Approach for Recommendation to Large Homogeneous and Automatically Detected Groups
Bobadilla, Jesús; Gutiérrez, Abraham; Alonso, Santiago; Hurtado, Remigio (International Journal of Interactive Multimedia and Artificial Intelligence (IJIMAI), 06/2020)In the collaborative filtering recommender systems (CFRS) field, recommendation to group of users is mainly focused on stablished, occasional or random groups. These groups have a little number of users: relatives, friends, ... -
Neural Collaborative Filtering Classification Model to Obtain Prediction Reliabilities
Bobadilla, Jesús; Gutiérrez, Abraham; Alonso, Santiago; González-Prieto, Ángel (International Journal of Interactive Multimedia and Artificial Intelligence (IJIMAI), 06/2022)Neural collaborative filtering is the state of art field in the recommender systems area; it provides some models that obtain accurate predictions and recommendations. These models are regression-based, and they just return ...