Neural Collaborative Filtering Classification Model to Obtain Prediction Reliabilities
Autor:
Bobadilla, Jesús
; Gutiérrez, Abraham
; Alonso, Santiago
; González-Prieto, Ángel
Fecha:
06/2022Palabra clave:
Revista / editorial:
International Journal of Interactive Multimedia and Artificial Intelligence (IJIMAI)Tipo de Ítem:
articleDirección web:
https://www.ijimai.org/journal/bibcite/reference/2997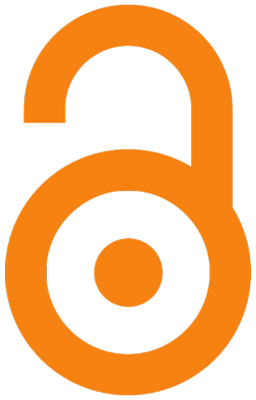
Resumen:
Neural collaborative filtering is the state of art field in the recommender systems area; it provides some models that obtain accurate predictions and recommendations. These models are regression-based, and they just
return rating predictions. This paper proposes the use of a classification-based approach, returning both rating predictions and their reliabilities. The extra information (prediction reliabilities) can be used in a variety of
relevant collaborative filtering areas such as detection of shilling attacks, recommendations explanation or navigational tools to show users and items dependences. Additionally, recommendation reliabilities can be
gracefully provided to users: “probably you will like this film”, “almost certainly you will like this song”, etc. This paper provides the proposed neural architecture; it also tests that the quality of its recommendation results is as good as the state of art baselines. Remarkably, individual rating predictions are improved by using the proposed architecture compared to baselines. Experiments have been performed making use of four popular public datasets, showing generalizable quality results. Overall, the proposed architecture improves individual rating predictions quality, maintains recommendation results and opens the doors to a set of relevant collaborative filtering fields.
Ficheros en el ítem
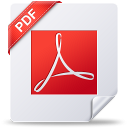
Este ítem aparece en la(s) siguiente(s) colección(es)
Estadísticas de uso
Año |
2012 |
2013 |
2014 |
2015 |
2016 |
2017 |
2018 |
2019 |
2020 |
2021 |
2022 |
2023 |
2024 |
2025 |
Vistas |
0 |
0 |
0 |
0 |
0 |
0 |
0 |
0 |
0 |
0 |
92 |
104 |
103 |
25 |
Descargas |
0 |
0 |
0 |
0 |
0 |
0 |
0 |
0 |
0 |
0 |
33 |
36 |
152 |
13 |
Ítems relacionados
Mostrando ítems relacionados por Título, autor o materia.
-
A Collaborative Filtering Probabilistic Approach for Recommendation to Large Homogeneous and Automatically Detected Groups
Bobadilla, Jesús; Gutiérrez, Abraham; Alonso, Santiago; Hurtado, Remigio (International Journal of Interactive Multimedia and Artificial Intelligence (IJIMAI), 06/2020)In the collaborative filtering recommender systems (CFRS) field, recommendation to group of users is mainly focused on stablished, occasional or random groups. These groups have a little number of users: relatives, friends, ... -
Classification-based Deep Neural Network Architecture for Collaborative Filtering Recommender Systems
Bobadilla, Jesús; Ortega, Fernando; Gutiérrez, Abraham; Alonso, Santiago (International Journal of Interactive Multimedia and Artificial Intelligence (IJIMAI), 03/2020)This paper proposes a scalable and original classification-based deep neural architecture. Its collaborative filtering approach can be generalized to most of the existing recommender systems, since it just operates on the ... -
DeepFair: Deep Learning for Improving Fairness in Recommender Systems
Bobadilla, Jesús; Lara-Cabrera, Raúl; González-Prieto, Ángel; Ortega, Fernando (International Journal of Interactive Multimedia and Artificial Intelligence (IJIMAI), 06/2021)The lack of bias management in Recommender Systems leads to minority groups receiving unfair recommendations. Moreover, the trade-off between equity and precision makes it difficult to obtain recommendations that meet both ...