S-Divergence-Based Internal Clustering Validation Index
Autor:
Kumar Sharma, Krishna
; Seal, Ayan
; Yazidi, Anis
; Krejcar, Ondrej
Fecha:
12/2023Palabra clave:
Revista / editorial:
International Journal of Interactive Multimedia and Artificial IntelligenceCitación:
K. K. Sharma, A. Seal, A. Yazidi, O. Krejcar. S-Divergence-Based Internal Clustering Validation Index, International Journal of Interactive Multimedia and Artificial Intelligence, (2023), http://dx.doi.org/10.9781/ijimai.2023.10.001Tipo de Ítem:
articleDirección web:
https://www.ijimai.org/journal/bibcite/reference/3381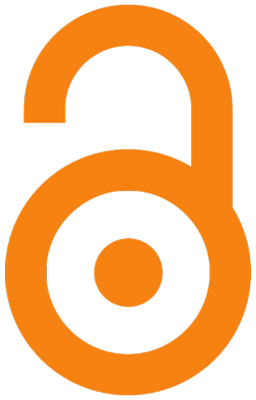
Resumen:
A clustering validation index (CVI) is employed to evaluate an algorithm’s clustering results. Generally, CVI statistics can be split into three classes, namely internal, external, and relative cluster validations. Most of the existing internal CVIs were designed based on compactness (CM) and separation (SM). The distance between cluster centers is calculated by SM, whereas the CM measures the variance of the cluster. However, the SM between groups is not always captured accurately in highly overlapping classes. In this article, we devise a novel internal CVI that can be regarded as a complementary measure to the landscape of available internal CVIs. Initially, a database’s clusters are modeled as a non-parametric density function estimated using kernel
density estimation. Then the S-divergence (SD) and S-distance are introduced for measuring the SM and the CM, respectively. The SD is defined based on the concept of Hermitian positive definite matrices applied to density functions. The proposed internal CVI (PM) is the ratio of CM to SM. The PM outperforms the legacy measures presented in the literature on both superficial and realistic databases in various scenarios, according to empirical results from four popular clustering algorithms, including fuzzy k-means, spectral clustering, density peak clustering, and density-based spatial clustering applied to noisy data.
Ficheros en el ítem
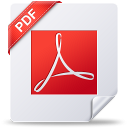
Este ítem aparece en la(s) siguiente(s) colección(es)
Estadísticas de uso
Año |
2012 |
2013 |
2014 |
2015 |
2016 |
2017 |
2018 |
2019 |
2020 |
2021 |
2022 |
2023 |
2024 |
Vistas |
0 |
0 |
0 |
0 |
0 |
0 |
0 |
0 |
0 |
0 |
0 |
41 |
121 |
Descargas |
0 |
0 |
0 |
0 |
0 |
0 |
0 |
0 |
0 |
0 |
0 |
30 |
40 |
Ítems relacionados
Mostrando ítems relacionados por Título, autor o materia.
-
Performance and Convergence Analysis of Modified C-Means Using Jeffreys-Divergence for Clustering
Seal, Ayan; Karlekar, Aditya; Krejcar, Ondrej; Herrera-Viedma, Enrique (International Journal of Interactive Multimedia and Artificial Intelligence (IJIMAI), 12/2021)The size of data that we generate every day across the globe is undoubtedly astonishing due to the growth of the Internet of Things. So, it is a common practice to unravel important hidden facts and understand the massive ... -
Imputation of Rainfall Data Using the Sine Cosine Function Fitting Neural Network
Chan Chiu, Po; Selamat, Ali; Krejcar, Ondrej; Kuok Kuok, King; Herrera-Viedma, Enrique; Fenza, Giuseppe (International Journal of Interactive Multimedia and Artificial Intelligence (IJIMAI), 09/2021)Missing rainfall data have reduced the quality of hydrological data analysis because they are the essential input for hydrological modeling. Much research has focused on rainfall data imputation. However, the compatibility ... -
Enhancing big data feature selection using a hybrid correlation-based feature selection
Mohamad, Masurah; Selamat, Ali; Krejcar, Ondrej; González-Crespo, Rubén ; Herrera-Viedma, Enrique; Fujita, Hamido (2021)This study proposes an alternate data extraction method that combines three well-known feature selection methods for handling large and problematic datasets: the correlation-based feature selection (CFS), best first search ...