Enhancing big data feature selection using a hybrid correlation-based feature selection
Autor:
Mohamad, Masurah
; Selamat, Ali
; Krejcar, Ondrej
; González-Crespo, Rubén
; Herrera-Viedma, Enrique
; Fujita, Hamido
Fecha:
2021Palabra clave:
Tipo de Ítem:
articleDirección web:
https://www.mdpi.com/2079-9292/10/23/2984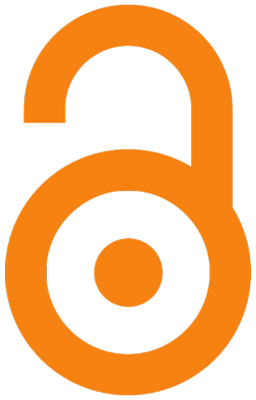
Resumen:
This study proposes an alternate data extraction method that combines three well-known feature selection methods for handling large and problematic datasets: the correlation-based feature selection (CFS), best first search (BFS), and dominance-based rough set approach (DRSA) methods. This study aims to enhance the classifier’s performance in decision analysis by eliminating uncorrelated and inconsistent data values. The proposed method, named CFS-DRSA, comprises several phases executed in sequence, with the main phases incorporating two crucial feature extraction tasks. Data reduction is first, which implements a CFS method with a BFS algorithm. Secondly, a data selection process applies a DRSA to generate the optimized dataset. Therefore, this study aims to solve the computational time complexity and increase the classification accuracy. Several datasets with various characteristics and volumes were used in the experimental process to evaluate the proposed method’s credibility. The method’s performance was validated using standard evaluation measures and benchmarked with other established methods such as deep learning (DL). Overall, the proposed work proved that it could assist the classifier in returning a significant result, with an accuracy rate of 82.1% for the neural network (NN) classifier, compared to the support vector machine (SVM), which returned 66.5% and 49.96% for DL. The one-way analysis of variance (ANOVA) statistical result indicates that the proposed method is an alternative extraction tool for those with difficulties acquiring expensive big data analysis tools and those who are new to the data analysis field.
Este ítem aparece en la(s) siguiente(s) colección(es)
Estadísticas de uso
Año |
2012 |
2013 |
2014 |
2015 |
2016 |
2017 |
2018 |
2019 |
2020 |
2021 |
2022 |
2023 |
2024 |
Vistas |
0 |
0 |
0 |
0 |
0 |
0 |
0 |
0 |
0 |
0 |
38 |
37 |
32 |
Descargas |
0 |
0 |
0 |
0 |
0 |
0 |
0 |
0 |
0 |
0 |
0 |
0 |
0 |
Ítems relacionados
Mostrando ítems relacionados por Título, autor o materia.
-
Multilayer Framework for Botnet Detection Using Machine Learning Algorithms
Ibrahim, Wan Nur Hidayah; Anuar, Syahid; Selamat, Ali; Krejcar, Ondrej; González-Crespo, Rubén; Herrera-Viedma, Enrique; Fujita, Hamido (IEEE Access, 2021)A botnet is a malware program that a hacker remotely controls called a botmaster. Botnet can perform massive cyber-attacks such as DDOS, SPAM, click-fraud, information, and identity stealing. The botnet also can avoid being ... -
Imputation of Rainfall Data Using the Sine Cosine Function Fitting Neural Network
Chan Chiu, Po; Selamat, Ali; Krejcar, Ondrej; Kuok Kuok, King; Herrera-Viedma, Enrique; Fenza, Giuseppe (International Journal of Interactive Multimedia and Artificial Intelligence (IJIMAI), 09/2021)Missing rainfall data have reduced the quality of hydrological data analysis because they are the essential input for hydrological modeling. Much research has focused on rainfall data imputation. However, the compatibility ... -
Dealing with group decision-making environments that have a high amount of alternatives using card-sorting techniques
Morente-Molinera, Juan Antonio; Ríos Aguilar, Sergio ; González-Crespo, Rubén ; Herrera-Viedma, Enrique (Expert Systems with Applications, 01/08/2019)Due to the appearance of Web 2.0 technologies and smartphones, the amount of information available to carry out group decision-making processes has increased dramatically. Therefore, there is a need for group decision-making ...