Imputation of Rainfall Data Using the Sine Cosine Function Fitting Neural Network
Autor:
Chan Chiu, Po
; Selamat, Ali
; Krejcar, Ondrej
; Kuok Kuok, King
; Herrera-Viedma, Enrique
; Fenza, Giuseppe
Fecha:
09/2021Palabra clave:
Revista / editorial:
International Journal of Interactive Multimedia and Artificial Intelligence (IJIMAI)Tipo de Ítem:
articleDirección web:
https://www.ijimai.org/journal/bibcite/reference/3001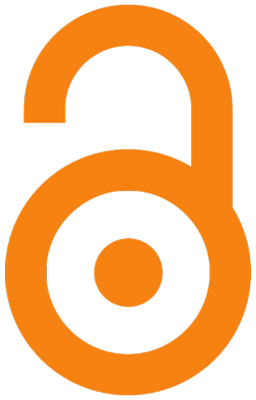
Resumen:
Missing rainfall data have reduced the quality of hydrological data analysis because they are the essential input for hydrological modeling. Much research has focused on rainfall data imputation. However, the compatibility of precipitation (rainfall) and non-precipitation (meteorology) as input data has received less attention. First, we propose a novel pre-processing mechanism for non-precipitation data by using principal component analysis (PCA). Before the imputation, PCA is used to extract the most relevant features from the meteorological data. The final output of the PCA is combined with the rainfall data from the nearest neighbor gauging stations and then used as the input to the neural network for missing data imputation. Second, a sine cosine algorithm is presented to optimize neural network for infilling the missing rainfall data. The proposed sine cosine function fitting neural network (SC-FITNET) was compared with the sine cosine feedforward neural network (SCFFNN), feedforward neural network (FFNN) and long short-term memory (LSTM) approaches. The results showed that the proposed SC-FITNET outperformed LSTM, SC-FFNN and FFNN imputation in terms of mean absolute error (MAE), root mean square error (RMSE) and correlation coefficient (R), with an average accuracy of 90.9%. This study revealed that as the percentage of missingness increased, the precision of the four imputation methods reduced. In addition, this study also revealed that PCA has potential in pre-processing meteorological data into an understandable format for the missing data imputation.
Ficheros en el ítem
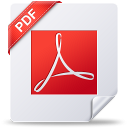
Este ítem aparece en la(s) siguiente(s) colección(es)
Estadísticas de uso
Año |
2012 |
2013 |
2014 |
2015 |
2016 |
2017 |
2018 |
2019 |
2020 |
2021 |
2022 |
2023 |
2024 |
Vistas |
0 |
0 |
0 |
0 |
0 |
0 |
0 |
0 |
0 |
0 |
50 |
118 |
173 |
Descargas |
0 |
0 |
0 |
0 |
0 |
0 |
0 |
0 |
0 |
0 |
17 |
37 |
82 |
Ítems relacionados
Mostrando ítems relacionados por Título, autor o materia.
-
Enhancing big data feature selection using a hybrid correlation-based feature selection
Mohamad, Masurah; Selamat, Ali; Krejcar, Ondrej; González-Crespo, Rubén ; Herrera-Viedma, Enrique; Fujita, Hamido (2021)This study proposes an alternate data extraction method that combines three well-known feature selection methods for handling large and problematic datasets: the correlation-based feature selection (CFS), best first search ... -
Multilayer Framework for Botnet Detection Using Machine Learning Algorithms
Ibrahim, Wan Nur Hidayah; Anuar, Syahid; Selamat, Ali; Krejcar, Ondrej; González-Crespo, Rubén; Herrera-Viedma, Enrique; Fujita, Hamido (IEEE Access, 2021)A botnet is a malware program that a hacker remotely controls called a botmaster. Botnet can perform massive cyber-attacks such as DDOS, SPAM, click-fraud, information, and identity stealing. The botnet also can avoid being ... -
Performance and Convergence Analysis of Modified C-Means Using Jeffreys-Divergence for Clustering
Seal, Ayan; Karlekar, Aditya; Krejcar, Ondrej; Herrera-Viedma, Enrique (International Journal of Interactive Multimedia and Artificial Intelligence (IJIMAI), 12/2021)The size of data that we generate every day across the globe is undoubtedly astonishing due to the growth of the Internet of Things. So, it is a common practice to unravel important hidden facts and understand the massive ...