Optimising Health Emergency Resource Management from Multi-Model Databases
Autor:
Arias, J.C.
; Cubillas, Juan Jose
; Ramos, Maria I.
Fecha:
2022Palabra clave:
Revista / editorial:
Electronics (Switzerland)Citación:
Arias, J. C., Cubillas, J. J., & Ramos, M. I. (2022). Optimising Health Emergency Resource Management from Multi-Model Databases. Electronics, 11(21), 3602. MDPI AG. Retrieved from http://dx.doi.org/10.3390/electronics11213602Tipo de Ítem:
Articulo Revista IndexadaDirección web:
https://www.mdpi.com/2079-9292/11/21/3602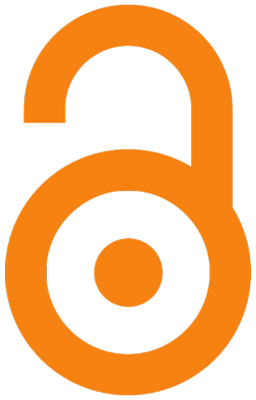
Resumen:
The health care sector is one of the most sensitive sectors in our society, and it is believed that the application of specific and detailed database creation and design techniques can improve the quality of patient care. In this sense, better management of emergency resources should be achieved. The development of a methodology to manage and integrate a set of data from multiple sources into a centralised database, which ensures a high quality emergency health service, is a challenge. The high level of interrelation between all of the variables related to patient care will allow one to analyse and make the right strategic decisions about the type of care that will be needed in the future, efficiently managing the resources involved in such care. An optimised database was designed that integrated and related all aspects that directly and indirectly affected the emergency care provided in the province of Jaén (city of Jaén, Andalusia, Spain) over the last eight years. Health, social, economic, environmental, and geographical information related to each of these emergency services was stored and related. Linear and nonlinear regression algorithms were used: support vector machine (SVM) with linear kernel and generated linear model (GLM), and the nonlinear SVM with Gaussian kernel. Predictive models of emergency demand were generated with a success rate of over 90%.
Ficheros en el ítem
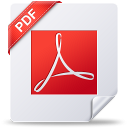
Nombre: optimising_health_emergency_resource_management_from_multimodel_databases.pdf
Tamaño: 2.032Mb
Formato: application/pdf
Este ítem aparece en la(s) siguiente(s) colección(es)
Estadísticas de uso
Año |
2012 |
2013 |
2014 |
2015 |
2016 |
2017 |
2018 |
2019 |
2020 |
2021 |
2022 |
2023 |
2024 |
Vistas |
0 |
0 |
0 |
0 |
0 |
0 |
0 |
0 |
0 |
0 |
0 |
23 |
20 |
Descargas |
0 |
0 |
0 |
0 |
0 |
0 |
0 |
0 |
0 |
0 |
0 |
7 |
32 |
Ítems relacionados
Mostrando ítems relacionados por Título, autor o materia.
-
Optimización de la calidad asistencial de urgencias y emergencias mediante bases de datos multidisciplinares
Arias, J.C.; Cubillas, Juan Jose; Ramos, Maria I.; Feito, F.R. (Iberian Conference on Information Systems and Technologies, CISTI, 2022)En la actualidad una de las técnicas más importantes para mejorar la calidad en la asistencia del paciente es el análisis exhaustivo de todos los aspectos de dicha asistencia mediante la utilización de bases de datos ... -
A Machine Learning Model for Early Prediction of Crop Yield, Nested in a Web Application in the Cloud: A Case Study in an Olive Grove in Southern Spain
Cubillas, Juan Jose; Ramos, Maria I.; Jurado, Juan Manuel; Feito, F.R. (Agriculture, 2022)Predictive systems are a crucial tool in management and decision-making in any productive sector. In the case of agriculture, it is especially interesting to have advance information on the profitability of a farm. In this ... -
Use of Data Mining to Predict the Influx of Patients to Primary Healthcare Centres and Construction of an Expert System
Cubillas, Juan Jose; Ramos, Maria I.; Feito, F.R. (Applied Sciences (Switzerland), 2022)In any productive sector, predictive tools are crucial for optimal management and decision-making. In the health sector, it is especially important to have information available in advance, as this not only means optimizing ...