Enhancing Tennis Serve Scoring Efficiency: An AI Deep Learning Approach
Autor:
Liu, Jing-Wei
Fecha:
07/2024Palabra clave:
Revista / editorial:
International Journal of Interactive Multimedia and Artificial Intelligence (IJIMAI)Citación:
J. W. Liu. Enhancing Tennis Serve Scoring Efficiency: An AI Deep Learning Approach, International Journal of Interactive Multimedia and Artificial Intelligence, (2024), http://dx.doi.org/10.9781/ijimai.2024.07.003Tipo de Ítem:
article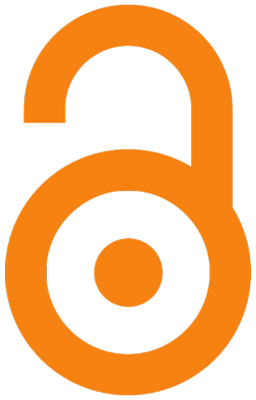
Resumen:
The playing field of a tennis competition is a dynamic and complex formative environment given the following preliminary knowledge: (a) the basic technical, tactical, situational, and special types of shots used by the opponent; (b) the hitting area of the tennis player; (c) the place of service; (d) the ball drop position; and (d) batting efficiency and other related information that may improve the chances of victory. In this study, we propose an AI classification model for tennis serve scores. Using a deep learning algorithm, the model automatically tracks and classifies the serve scores of professional tennis players from video data. We first defined the players’ techniques, volleys, and placements of strokes and serves. Subsequently, we defined the referee's tennis terms and the voice in deciding on a serve score. Finally, we developed a deep learning model to automatically classify the serving position, landing position, and use of tennis techniques. The methodology was applied in the context of 10 matches played by Roger Federer and Rafael Nadal. The proposed deep learning algorithm achieved a 98.27% accuracy in the automatic classification of serve scores, revealing that Nadal outscored Federer by 2.1% in terms of serve-scoring efficiency. These results are expected to facilitate the automatic comparison and classification of shots in future studies, enabling coaches to adjust tactics in a timely manner and thereby improve the chances of winning.
Ficheros en el ítem
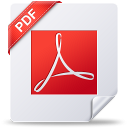
Nombre: Enhancing Tennis Serve Scoring Efficiency.pdf
Tamaño: 591.4Kb
Formato: application/pdf
Este ítem aparece en la(s) siguiente(s) colección(es)
Estadísticas de uso
Año |
2012 |
2013 |
2014 |
2015 |
2016 |
2017 |
2018 |
2019 |
2020 |
2021 |
2022 |
2023 |
2024 |
2025 |
Vistas |
0 |
0 |
0 |
0 |
0 |
0 |
0 |
0 |
0 |
0 |
0 |
0 |
134 |
37 |
171 |
Descargas |
0 |
0 |
0 |
0 |
0 |
0 |
0 |
0 |
0 |
0 |
0 |
0 |
66 |
45 |
111 |
Ítems relacionados
Mostrando ítems relacionados por Título, autor o materia.
-
The Human Motion Behavior Recognition by Deep Learning Approach and the Internet of Things
Li, Hui; Liu, Huayang; Zhao, Wei; Liu, Hao (International Journal of Interactive Multimedia and Artificial Intelligence (IJIMAI), 07/2024)This paper is dedicated to exploring the practical implementation of deep learning and Internet of Things (IoT) technology within systems designed for recognizing human motion behavior. It places a particular emphasis on ... -
Research on Brain and Mind Inspired Intelligence
Liu, Yang; Wei, Jianshe (International Journal of Interactive Multimedia and Artificial Intelligence, 12/2023)To address the problems of scientific theory, common technology and engineering application of multimedia and multimodal information computing, this paper is focused on the theoretical model, algorithm framework, and system ... -
Inadequate dataset learning for major depressive disorder MRI semantic classification
Liu, Jie; Dey, Nilanjan; González-Crespo, Rubén ; Shi, Fuqian; Liu, Chanjuan (IET Image Processing, 2022)Predicting patients with major depression (MDD) is currently a difficult task. Magnetic resonance imaging (MRI) data analysis may provide insight into individual patient responses, allowing for more customized treatment ...