Comparativa U-Net vs. ResNet + PsPNet para el dataset A2D2.
Autor:
Justo-Sarmentero, Luis Vicente
Fecha:
08/11/2023Palabra clave:
Tipo de Ítem:
masterThesis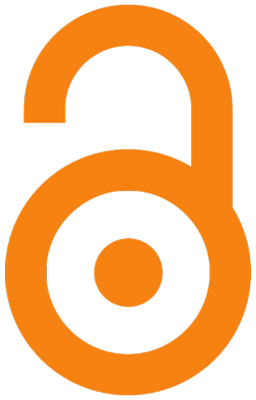
Resumen:
Los avances en el desarrollo y comercialización de sistemas de
conducción autónoma han tenido una fuerte aceleración en los últimos
años. La complejidad de estos sistemas, la cantidad y diversidad de datos
que utilizan y la velocidad de respuesta que necesitan, obligan a buscar
soluciones con la máxima velocidad ejecución y el menor coste
computacional posible. Dentro de esta búsqueda, la experimentación y
comparación de diferentes alternativas para la interpretación del entorno
del vehículo es capital para encontrar la solución óptima. Este trabajo se
centra en la tarea de la visión artificial para la interpretación del entorno y
particularmente en la segmentación semántica de imágenes mediante la
utilización de redes neuronales U-Net, ResNet y PsPNet.
El objetivo de este trabajo es contribuir a encontrar la solución más óptima
para la tarea de segmentación semántica realizando el entrenamiento, con
el dataset A2D2 de Audi AG®, de una red neuronal basada en la
arquitectura U-Net y la comparación de su rendimiento con el obtenido por
los autores de la publicación del mencionado dataset. Dichos autores,
utilizaron una arquitectura compuesta por la combinación de las redes
neuronales ResNet y PsPNet.
En este proyecto, primero se abordan los enfoques actuales para la
conducción autónoma y cómo encaja la segmentación semántica de
imágenes en ellos. Se desarrollan los elementos principales para la
ejecución de esta tarea como sensores, dataset, simuladores,
herramientas ETL y técnicas de inteligencia artificial profundizando en los
distintos tipos de redes neuronales. Se proporciona un método completo
mediante la utilización de librerías de Python, TensorFlow y Keras para la
extracción, tratamiento y carga del dataset A2D2, la construcción del
modelo de red neuronal basado en U-Net, su entrenamiento con dicho
dataset, y la evaluación del mismo bajo las métricas más comunes en
segmentación semántica.
Como resultado de este trabajo, además del análisis en profundidad del
dataset A2D2 y los algoritmos en Python comentados al detalle, se obtiene
el rendimiento del modelo U-Net para los índices Dice e IoU, así como el
tiempo de entrenamiento y evaluación. Estos resultados se comparan con
los publicados por los autores del dataset A2D2 de Audi AG® .
Esta comparación permite concluir que la arquitectura U-Net no presenta
una mejora en el rendimiento con respecto a los resultados obtenidos por
los mencionados autores. No obstante, dado que los valores de tiempo de
entrenamiento y tiempo de valuación no han sido publicados por dichos
autores no es posible compararlos con los obtenidos en este trabajo, por
lo que quedan disponibles para posibles líneas de trabajo futuras. De igual
modo, como futuros trabajos, se propone la parametrización de la red
neuronal U-Net de forma diferente para conseguir una mejora del
rendimiento.
Descripción:
Advances in the development and commercialization of autonomous
driving systems have experienced a significant acceleration in recent
years. The complexity of these systems, the volume and diversity of data
they utilize, and the speed of response they require compel the search for
solutions that offer maximal execution speed and minimal computational
cost. Within this pursuit, experimentation and comparison of different
alternatives for vehicle environment interpretation are crucial in finding the
optimal solution. This work focuses on the task of artificial vision for
environment interpretation, particularly on semantic image segmentation
using U-Net, ResNet, and PsPNet neural networks.
The objective of this study is to contribute to finding the optimal solution for
semantic segmentation tasks by training a neural network based on the UNet
architecture with the A2D2 from Audi AG ® dataset. The performance
of this model is then compared with the results obtained by the authors of
the aforementioned dataset publication. These authors employed a
combination of ResNet and PsPNet neural networks for their architecture.
In this project, we first address the current approaches for autonomous
driving and how semantic image segmentation fits into them. The main
elements for the execution of this task such as sensors, datasets,
simulators, ETL tools and artificial intelligence techniques are developed
by delving into the different types of neural networks. A complete method
is provided using Python and TensorFlow libraries for the extraction,
processing and loading of the A2D2 dataset as well as for the construction
of the neural network model based on U-Net, its training with this dataset,
and its evaluation under the most common metrics in semantic
segmentation.
Luis Vicente Justo Sarmentero Máster Universitario en Análisis y Visualización de Datos Masivos
Comparativa U-Net vs. ResNet + PsPNet para dataset A2D2 4
As a result of this work, along with an in-depth analysis of the A2D2 dataset
and extensively commented Python algorithms, the performance of the UNet
model is obtained in terms of Dice and IoU indices, as well as training
and evaluation times. These results are compared with those published by
the authors of the A2D2 dataset from Audi AG® .
This comparison leads to the conclusion that the U-Net architecture does
not exhibit improved performance compared to the results achieved by the
aforementioned authors. However, since the training and evaluation time
values have not been published by said authors, a comparison with the
results of this work is not feasible. Therefore, these aspects remain
available for potential future lines of work. Similarly, future work could
involve parameterizing
Ficheros en el ítem
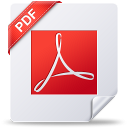
Este ítem aparece en la(s) siguiente(s) colección(es)
Estadísticas de uso
Año |
2012 |
2013 |
2014 |
2015 |
2016 |
2017 |
2018 |
2019 |
2020 |
2021 |
2022 |
2023 |
2024 |
2025 |
Vistas |
0 |
0 |
0 |
0 |
0 |
0 |
0 |
0 |
0 |
0 |
0 |
29 |
361 |
113 |
503 |
Descargas |
0 |
0 |
0 |
0 |
0 |
0 |
0 |
0 |
0 |
0 |
0 |
9 |
119 |
25 |
153 |
Ítems relacionados
Mostrando ítems relacionados por Título, autor o materia.
-
Linked Data Methodologies for Managing Information about Television Content
Redondo-García, José Luis; Botón-Fernández, Vicente; Lozano-Tello, Adolfo (International Journal of Interactive Multimedia and Artificial Intelligence (IJIMAI), 09/2012)OntoTV is a television information management system designed for improving the quality and quantity of the information available in the current television platforms. In order to achieve this objective, OntoTV (1) collects ... -
HIV co-infection in HTLV-1 carriers in Spain
Mendoza, Carmen de; Caballero, Estrella; Aguilera, Antonio; Benito, Rafael; Maciá, Dolores; García-Costa, Juan; Soriano, Vicente ; Corral, Octavio Jorge ; Gómez-Gallego, Felix ; ...et al.; Morano, Luis (Virus Research, 06/2019)Background: Human retroviruses HIV and HTLV share transmission routes. HIV widely spread in Spain during the 80 s through injection drug use and sex, and nowadays HIV rates in Spain account for one of the largest in Europe. ... -
Predictors of Hepatitis C Treatment Failure After Using Direct-Acting Antivirals in People Living With Human Immunodeficiency Virus
Cachay, Edward R.; Mena, Alvaro; Morano, Luis; Benitez, Laura; Maida, Ivana; Ballard, Craig; Soriano, Vicente ; ...et al.; Mathews, Wm. C. (Open Forum Infectious Diseases, 03/2019)Background. Little is known about the influence of ongoing barriers to care in the persistence of hepatitis C virus (HCV) viremia after treatment with direct-acting antivirals (DAAs) among people living with human ...