An Improved Deep Learning Model for Electricity Price Forecasting
Autor:
Iqbal, Rashed
; Mokhlis, Hazlie
; Mohd Khairuddin, Anis Salwa
; Azam Muhammad, Munir
Fecha:
06/2023Palabra clave:
Revista / editorial:
International Journal of Interactive Multimedia and Artificial IntelligenceTipo de Ítem:
articleDirección web:
https://www.ijimai.org/journal/bibcite/reference/3327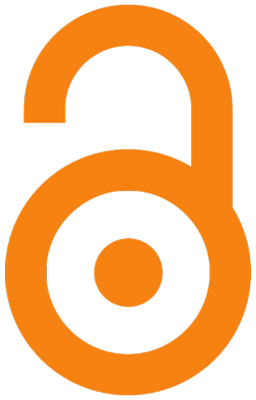
Resumen:
Accurate electricity price forecasting (EPF) is important for the purpose of bidding strategies and minimizing the risk for market participants in the competitive electricity market. Besides that, EPF becomes critically important for effective planning and efficient operation of a power system due to deregulation of electricity industry. However, accurate EPF is very challenging due to complex nonlinearity in the time series-based electricity prices. Hence, this work proposed two-fold contributions which are (1) effective time series preprocessing module to ensure feasible time-series data is fitted in the deep learning model, and (2) an improved long short-term memory (LSTM) model by incorporating linear scaled hyperbolic tangent (LiSHT) layer in the EPF. In this work, the time series pre-processing module adopted linear trend of the correlated features of electricity price series and the time series are tested by using Augmented Dickey Fuller (ADF) test method. In addition, the time series are transformed using boxcox transformation method in order to satisfy the stationarity property. Then, an improved LSTM prediction module is proposed to forecast electricity prices where LiSHT layer is adopted to optimize the parameters of the heterogeneous LSTM. This study is performed using the Australian electricity market price, load and renewable energy supply data. The experimental results obtained show that the proposed EPF framework performed better compared to previous techniques.
Ficheros en el ítem
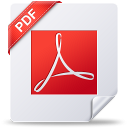
6 readers on Mendeley
Este ítem aparece en la(s) siguiente(s) colección(es)
Estadísticas de uso
Año |
2012 |
2013 |
2014 |
2015 |
2016 |
2017 |
2018 |
2019 |
2020 |
2021 |
2022 |
2023 |
2024 |
2025 |
Vistas |
0 |
0 |
0 |
0 |
0 |
0 |
0 |
0 |
0 |
0 |
0 |
54 |
198 |
45 |
297 |
Descargas |
0 |
0 |
0 |
0 |
0 |
0 |
0 |
0 |
0 |
0 |
0 |
73 |
74 |
23 |
170 |
Ítems relacionados
Mostrando ítems relacionados por Título, autor o materia.
-
Genetic Algorithm for Restricted Maximum k-Satisfiability in the Hopfield Network
Kasihmuddin, Mohd Shareduwan Bin Mohd; Mansor, Mohd Asyraf Bin; Sathasivam, Saratha (International Journal of Interactive Multimedia and Artificial Intelligence (IJIMAI), 12/2016)The restricted Maximum k-Satisfiability MAX- kSAT is an enhanced Boolean satisfiability counterpart that has attracted numerous amount of research. Genetic algorithm has been the prominent optimization heuristic algorithm ... -
Robust Artificial Immune System in the Hopfield network for Maximum k-Satisfiability
Bin Mohd Kasihmuddin, Mohd Shareduwan; Bin Mansor, Mohd Asyraf; Sathasivam, Saratha (International Journal of Interactive Multimedia and Artificial Intelligence (IJIMAI), 06/2017)Artificial Immune System (AIS) algorithm is a novel and vibrant computational paradigm, enthused by the biological immune system. Over the last few years, the artificial immune system has been sprouting to solve numerous ... -
Satisfiability Logic Analysis Via Radial Basis Function Neural Network with Artificial Bee Colony Algorithm
Kasihmuddin, Mohd Shareduwan Bin Mohd; Mansor, Mohd Asyraf Bin; Abdulhabib Alzaeemi, Shehab; Sathasivam, Saratha (International Journal of Interactive Multimedia and Artificial Intelligence (IJIMAI), 06/2021)Radial Basis Function Neural Network (RBFNN) is a variant of artificial neural network (ANN) paradigm, utilized in a plethora of fields of studies such as engineering, technology and science. 2 Satisfiability (2SAT) ...