A Spatio-Temporal Attention Graph Convolutional Networks for Sea Surface Temperature Prediction
Autor:
Chen, Desheng
; Wen, Jiabao
; Lv, Caiyun
Fecha:
03/2023Palabra clave:
Revista / editorial:
International Journal of Interactive Multimedia and Artificial Intelligence (IJIMAI)Tipo de Ítem:
articleDirección web:
https://www.ijimai.org/journal/bibcite/reference/3280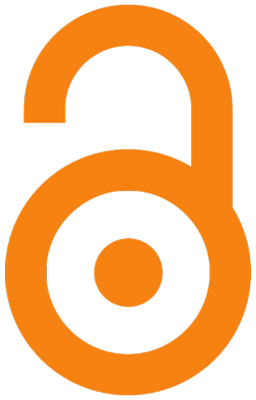
Resumen:
Sea surface temperature (SST) is an important index to detect ocean changes, predict SST anomalies, and prevent natural disasters caused by abnormal changes, dynamic variation of which have a profound impact on the whole marine ecosystem and the dynamic changes of climate. In order to better capture the dynamic changes of ocean temperature, it’s vitally essential to predict the SST in the future. A new spatio-temporal attention graph convolutional network (STAGCN) for SST prediction was proposed in this paper which can capture spatial dependence and temporal correlation in the way of integrating gated recurrent unit (GRU) model with graph convolutional network (GCN) and introduced attention mechanism. The STAGCN model adopts the GCN model to learn the topological structure between ocean location points for extracting the spatial characteristics from the ocean position nodes network. Besides, capturing temporal correlation by learning dynamic variation of SST time series data, a GRU model is introduced into the STAGCN model to deal with the prediction problem about long time series, the input of which is the SST data with spatial characteristics. To capture the significance of SST information at different times and increase the accuracy of SST forecast, the attention mechanism was used to obtain the spatial and temporal characteristics globally. In this study, the proposed STAGCN model was trained and tested on the East China Sea. Experiments with different prediction lengths show that the model can capture the spatio-temporal correlation of regional-scale sea surface temperature series and almost uniformly outperforms other classical models under different sea areas and different prediction levels, in which the root mean square error is reduced by about 0.2 compared with the LSTM model.
Ficheros en el ítem
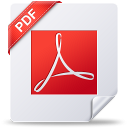
Este ítem aparece en la(s) siguiente(s) colección(es)
Estadísticas de uso
Año |
2012 |
2013 |
2014 |
2015 |
2016 |
2017 |
2018 |
2019 |
2020 |
2021 |
2022 |
2023 |
2024 |
Vistas |
0 |
0 |
0 |
0 |
0 |
0 |
0 |
0 |
0 |
0 |
0 |
124 |
166 |
Descargas |
0 |
0 |
0 |
0 |
0 |
0 |
0 |
0 |
0 |
0 |
0 |
103 |
61 |
Ítems relacionados
Mostrando ítems relacionados por Título, autor o materia.
-
Preface
Pang, C.; Chen, G.; Chen, L.; Zhang, B.; Li, Q.; Gao, Y.; Popescu, E.; Hao, T.; Navarro, S.M.B. ; Klamma, R. (Lecture notes in computer science (including subseries lecture notes in artificial intelligence and lecture notes in bioinformatics), 2021)Preface -
An Ensemble Classifier for Stock Trend Prediction Using Sentence-Level Chinese News Sentiment and Technical Indicators
Chen, Chun-Hao; Chen, Po-Yeh; Chun-Wei Lin, Jerry (International Journal of Interactive Multimedia and Artificial Intelligence (IJIMAI), 03/2022)In the financial market, predicting stock trends based on stock market news is a challenging task, and researchers are devoted to developing forecasting models. From the existing literature, the performance of the forecasting ... -
Modified YOLOv4-DenseNet Algorithm for Detection of Ventricular Septal Defects in Ultrasound Images
Chen, Shih-Hsin; Wang, Chun-Wei; Tai, I-Hsin; Weng, Ken-Pen; Chen, Yi-Hui; Hsieh, Kai-Sheng (International Journal of Interactive Multimedia and Artificial Intelligence (IJIMAI), 09/2021)Doctors conventionally analyzed echocardiographic images for diagnosing congenital heart diseases (CHDs). However, this process is laborious and depends on the experience of the doctors. This study investigated the use of ...