Electromiographic Signal Processing Using Embedded Artificial Intelligence: An Adaptive Filtering Approach
Autor:
Proaño-Guevara, Daniel
; Blanco Valencia, Xiomara Patricia
; Rosero-Montalvo, Paul D.
; Peluffo-Ordóñez, Diego H.
Fecha:
09/2022Palabra clave:
Revista / editorial:
International Journal of Interactive Multimedia and Artificial Intelligence (IJIMAI)Tipo de Ítem:
articleDirección web:
https://www.ijimai.org/journal/bibcite/reference/3162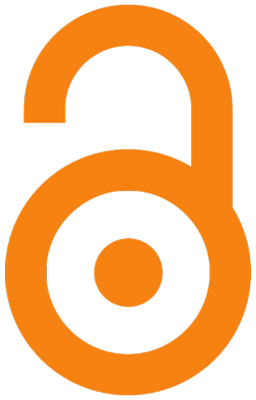
Resumen:
In recent times, Artificial Intelligence (AI) has become ubiquitous in technological fields, mainly due to its ability to perform computations in distributed systems or the cloud. Nevertheless, for some applications -as the case of EMG signal processing- it may be highly advisable or even mandatory an on-the-edge processing, i.e., an embedded processing methodology. On the other hand, sEMG signals have been traditionally processed using LTI techniques for simplicity in computing. However, making this strong assumption leads to information loss and spurious results. Considering the current advances in silicon technology and increasing computer power, it is possible to process these biosignals with AI-based techniques correctly. This paper presents an embedded-processing-based adaptive filtering system (here termed edge AI) being an outstanding alternative in contrast to a sensor-computer- actuator system and a classical digital signal processor (DSP) device. Specifically, a PYNQ-Z1 embedded system is used. For experimental purposes, three methodologies on similar processing scenarios are compared. The results show that the edge AI methodology is superior to benchmark approaches by reducing the processing time compared to classical DSPs and general standards while maintaining the signal integrity and processing it, considering that the EMG system is not LTI. Likewise, due to the nature of the proposed architecture, handling information exhibits no leakages. Findings suggest that edge computing is suitable for EMG signal processing when an on-device analysis is required.
Ficheros en el ítem
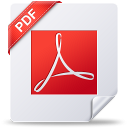
Este ítem aparece en la(s) siguiente(s) colección(es)
Estadísticas de uso
Año |
2012 |
2013 |
2014 |
2015 |
2016 |
2017 |
2018 |
2019 |
2020 |
2021 |
2022 |
2023 |
2024 |
Vistas |
0 |
0 |
0 |
0 |
0 |
0 |
0 |
0 |
0 |
0 |
32 |
128 |
120 |
Descargas |
0 |
0 |
0 |
0 |
0 |
0 |
0 |
0 |
0 |
0 |
28 |
135 |
52 |
Ítems relacionados
Mostrando ítems relacionados por Título, autor o materia.
-
Editor's Note
Blanco Valencia, Xiomara Patricia (International Journal of Interactive Multimedia and Artificial Intelligence (IJIMAI), 12/2021)The International Journal of Interactive Multimedia and Artificial Intelligence - IJIMAI - provides a space in which scientists and professionals can report about new advances in Artificial Intelligence (AI). On this ... -
Semiautomatic Grading of Short Texts for Open Answers in Higher Education
de-la-Fuente-Valentín, Luis ; Verdú, Elena ; Padilla-Zea, Natalia ; Villalonga, Claudia; Blanco Valencia, Xiomara Patricia ; Baldiris, Silvia (Communications in Computer and Information Science, 2022)Grading student activities in online courses is a time-expensive task, especially with a high number of students in the course. To avoid a bottleneck in the continuous evaluation process, quizzes with multiple choice ... -
Automated segmentation of leukocyte from hematological images—a study using various CNN schemes
Kadry, Seifedine; Rajinikanth, Venkatesan; Taniar, David; Damaševičius, Roberta; Blanco Valencia, Xiomara Patricia (Springer, 2022)Medical images play a fundamental role in disease screening, and automated evaluation of these images is widely preferred in hospitals. Recently, Convolutional Neural Network (CNN) supported medical data assessment is ...