Efficient and Robust Model Benchmarks with Item Response Theory and Adaptive Testing
Autor:
Song, Hao
; Flach, Peter
Fecha:
03/2021Palabra clave:
Revista / editorial:
International Journal of Interactive Multimedia and Artificial Intelligence (IJIMAI)Tipo de Ítem:
articleDirección web:
https://www.ijimai.org/journal/bibcite/reference/2901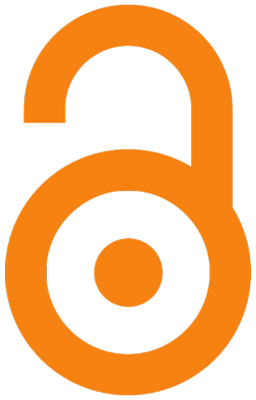
Resumen:
Progress in predictive machine learning is typically measured on the basis of performance comparisons on benchmark datasets. Traditionally these kinds of empirical evaluation are carried out on large numbers of datasets, but this is becoming increasingly hard due to computational requirements and the often large number of alternative methods to compare against. In this paper we investigate adaptive approaches to achieve better efficiency on model benchmarking. For a large collection of datasets, rather than training and testing a given approach on every individual dataset, we seek methods that allow us to pick only a few representative datasets to quantify the model’s goodness, from which to extrapolate to performance on other datasets. To this end, we adapt existing approaches from psychometrics: specifically, Item Response Theory and Adaptive Testing. Both are well-founded frameworks designed for educational tests. We propose certain modifications following the requirements of machine learning experiments, and present experimental results to validate the approach.
Ficheros en el ítem
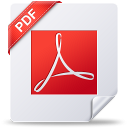
Este ítem aparece en la(s) siguiente(s) colección(es)
Estadísticas de uso
Año |
2012 |
2013 |
2014 |
2015 |
2016 |
2017 |
2018 |
2019 |
2020 |
2021 |
2022 |
2023 |
2024 |
Vistas |
0 |
0 |
0 |
0 |
0 |
0 |
0 |
0 |
0 |
0 |
31 |
63 |
119 |
Descargas |
0 |
0 |
0 |
0 |
0 |
0 |
0 |
0 |
0 |
0 |
18 |
35 |
54 |
Ítems relacionados
Mostrando ítems relacionados por Título, autor o materia.
-
Preface
Pang, C.; Chen, G.; Chen, L.; Zhang, B.; Li, Q.; Gao, Y.; Popescu, E.; Hao, T.; Navarro, S.M.B. ; Klamma, R. (Lecture notes in computer science (including subseries lecture notes in artificial intelligence and lecture notes in bioinformatics), 2021)Preface -
An Ensemble Classifier for Stock Trend Prediction Using Sentence-Level Chinese News Sentiment and Technical Indicators
Chen, Chun-Hao; Chen, Po-Yeh; Chun-Wei Lin, Jerry (International Journal of Interactive Multimedia and Artificial Intelligence (IJIMAI), 03/2022)In the financial market, predicting stock trends based on stock market news is a challenging task, and researchers are devoted to developing forecasting models. From the existing literature, the performance of the forecasting ... -
A Generalized Wine Quality Prediction Framework by Evolutionary Algorithms
Hui-Ye Chiu, Terry; Wu, Chienwen; Chen, Chun-Hao (International Journal of Interactive Multimedia and Artificial Intelligence (IJIMAI), 09/2021)Wine is an exciting and complex product with distinctive qualities that makes it different from other manufactured products. Therefore, the testing approach to determine the quality of wine is complex and diverse. Several ...