A real-life machine learning experience for predicting university dropout at different stages using academic data
Autor:
Fernández-García, Antonio Jesús
; Preciado, Juan Carlos
; Melchor, Fran
; Rodriguez-Echeverría, Roberto
; Conejero Manzano, José María
; Sánchez, Fernando
Fecha:
2021Palabra clave:
Revista / editorial:
Institute of Electrical and Electronics Engineers Inc.Tipo de Ítem:
articleDirección web:
https://ieeexplore.ieee.org/document/9548895/authors#authors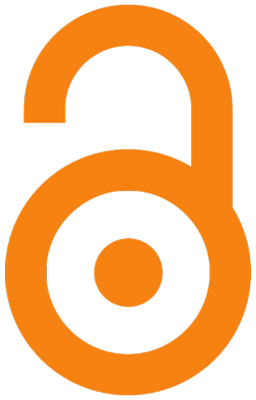
Resumen:
High levels of school dropout are a major burden on the educational and professional development of a country's inhabitants. A country's prosperity depends, among other factors, on its ability to produce higher education graduates capable of moving a country forward. To alleviate the dropout problem, more and more institutions are turning to the possibilities that artificial intelligence can provide to predict dropout as early as possible. The difficulty of accessing personal data and privacy issues that it entails force the institutions to rely on the Academic Data of their students to create accurate and reliable predictive systems. This work focuses on creating the best possible predictive model based solely on academic data, and accordingly, its capacity to infer knowledge must be maximised. Thus, Feature Engineering and Instance Engineering techniques such as dealing with redundancy, significance of the features, correlation, cardinality features, missing values, creation or elimination of features, data fusion, removal of unuseful instances, binning, resampling, normalisation, or encoding are applied in detail before the construction of well-known models such as Gradient Boosting, Random Forest, and Support Vector Machine along with an Ensemble of them at different stages: Prior to enrolment, at the end of the first semester, at the end of the second semester, at the end of the third semester, and at the end of the fourth semester. Through the construction of these predictive models that serve as inputs to a decision support system, the application of effective dropout prevention policies can be applied.
Este ítem aparece en la(s) siguiente(s) colección(es)
Estadísticas de uso
Año |
2012 |
2013 |
2014 |
2015 |
2016 |
2017 |
2018 |
2019 |
2020 |
2021 |
2022 |
2023 |
2024 |
2025 |
Vistas |
0 |
0 |
0 |
0 |
0 |
0 |
0 |
0 |
0 |
0 |
71 |
101 |
108 |
47 |
327 |
Descargas |
0 |
0 |
0 |
0 |
0 |
0 |
0 |
0 |
0 |
0 |
0 |
0 |
0 |
0 |
0 |
Ítems relacionados
Mostrando ítems relacionados por Título, autor o materia.
-
Creating a Recommender System to Support Higher Education Students in the Subject Enrollment Decision
Fernández-García, Antonio Jesús ; Rodríguez-Echeverría, Roberta; Preciado, Juan Carlos; Conejero Manzano, José María; Sánchez-Figueroa, Fernando (IEEE Access, 2020)Higher Education plays a principal role in the changing and complex world of today, and there has been rapid growth in the scientific literature dedicated to predicting students academic success or risk of dropout thanks ... -
Towards the use of Data Engineering, Advanced Visualization techniques and Association Rules to support knowledge discovery for public policies
Conejero Manzano, José María; Preciado, Juan Carlos; Fernández-García, Antonio Jesús ; Prieto, Álvaro; Rodriguez-Echeverría, Roberto (Expert systems with applications, 2021)Education and employment are key aspects of a country's well-being. Governments expend valuable resources on designing education plans and employment programs. These two aspects are usually analysed separately, although, ... -
A hybrid multidimensional Recommender System for radio programs
Fernández-García, Antonio Jesús ; Rodriguez-Echeverría, Roberto; Preciado, Juan Carlos; Perianez, Jorge; Gutiérrez, Juan D. (Expert Systems with Applications, 2022)The rise of Recommender Systems has made their presence very common today in many domains. An example is the domain of radio or TV broadcasting content recommendations. The approach proposed here allows radio listeners to ...