Evaluating the Impact of Pumping on Groundwater Level Prediction in the Chuoshui River Alluvial Fan Using Artificial Intelligence Techniques
Autor:
Su, Yu-Sheng
; Hu, Yu-Cheng
; Wu, Yun-Chin
; Lo, Ching-Teng
Fecha:
04/2024Palabra clave:
Revista / editorial:
International Journal of Interactive Multimedia and Artificial Intelligence (IJIMAI)Citación:
Y. S. Su, Y. C. Hu, Y. C. Wu, C. T. Lo. Evaluating the Impact of Pumping on Groundwater Level Prediction in the Chuoshui River Alluvial Fan Using Artificial Intelligence Techniques, International Journal of Interactive Multimedia and Artificial Intelligence, (2024), http://dx.doi. org/10.9781/ijimai.2024.04.002Tipo de Ítem:
article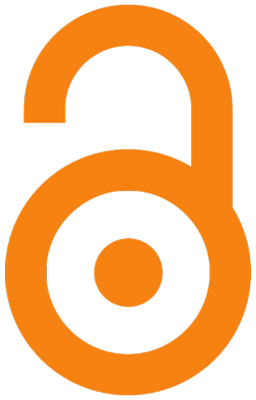
Resumen:
Over the past decade, excessive groundwater extraction has been the leading cause of land subsidence in Taiwan's Chuoshui River Alluvial Fan (CRAF) area. To effectively manage and monitor groundwater resources, assessing the effects of varying seasonal groundwater extraction on groundwater levels is necessary. This study focuses on the CRAF in Taiwan. We applied three artificial intelligence techniques for three predictive models: multiple linear regression (MLR), support vector regression (SVR), and Long Short-Term Memory Networks (LSTM). Each prediction model evaluated the extraction rate, considering temporal and spatial correlations. The study aimed to predict groundwater level variations by comparing the results of different models. This study used groundwater level and extraction data from the CRAF area in Taiwan. The dataset we constructed was the input variable for predicting groundwater level variations. The experimental results show that the LSTM method is the most suitable and stable deep learning model for predicting groundwater level variations in the CRAF, Taiwan, followed by the SVR method and finally the MLR method. Additionally, when considering different distances and depths of pumping data at groundwater level monitoring stations, it was found that the Guosheng and Hexing groundwater level monitoring stations are best predicted using pumping data within a distance of 20 kilometers and a depth of 20 meters.
Ficheros en el ítem
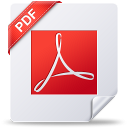
Nombre: Evaluating the Impact of Pumping on Groundwater Level Prediction in the Chuoshui River Alluvial Fan Using Artificial Intelligence Techniques.pdf
Tamaño: 2.612Mb
Formato: application/pdf
Este ítem aparece en la(s) siguiente(s) colección(es)
Estadísticas de uso
Año |
2012 |
2013 |
2014 |
2015 |
2016 |
2017 |
2018 |
2019 |
2020 |
2021 |
2022 |
2023 |
2024 |
2025 |
Vistas |
0 |
0 |
0 |
0 |
0 |
0 |
0 |
0 |
0 |
0 |
0 |
0 |
132 |
38 |
170 |
Descargas |
0 |
0 |
0 |
0 |
0 |
0 |
0 |
0 |
0 |
0 |
0 |
0 |
87 |
26 |
113 |
Ítems relacionados
Mostrando ítems relacionados por Título, autor o materia.
-
Introduction to the Special Section on Social Computing and Social Internet of Things
Hsu, Ching-Hsien; Montenegro Marin, Carlos Enrique; González-Crespo, Rubén ; Mohamed El-Sayed, Hassan Fouad (IEEE Transactions on Network Science and Engineering, 2022)The papers in this special section focus on social computing and the social Internet of Things (SIoT). SIoT is a new and latest paradigm that extends Internet of Things. This provides an ideal platform for interconnected ... -
A Framework for Extractive Text Summarization Based on Deep Learning Modified Neural Network Classifier
Muthu, Bala Anand; Cb, Sivaparthipan; Kumar, Priyan Malarvizhi; Kadry, Seifedine; Hsu, Ching-Hsien; Sanjuán Martínez, Óscar; González-Crespo, Rubén (Association for Computing Machinery, 2021)There is an exponential growth of text data over the internet, and it is expected to gain significant growth and attention in the coming years. Extracting meaningful insights from text data is crucially important as it ... -
Preface
Singh, Vijendra; Asari, Vijayan K.; Li, Kuan-Ching; González-Crespo, Rubén (Procedia Computer Science, 2022)We are pleased to present this special issue of Elsevier’s Procedia Computer Science Journal, which consists of the proceedings of the international conference on Machine Learning and Data Engineering (ICMLDE 2022).