Comparative Analysis of Building Insurance Prediction Using Some Machine Learning Algorithms
Autor:
Ejiyi, Chukwuebuka Joseph
; Qin, Zhen
; Salako, Abdulhaq Adetunji
; Happy, Monday Nkanta
; Nneji, Grace Ugochi
; Ukwuoma, Chiagoziem Chima
; Chikwendu, Ijeoma Amuche
; Gen, Ji
Fecha:
03/2022Palabra clave:
Revista / editorial:
International Journal of Interactive Multimedia and Artificial Intelligence (IJIMAI)Tipo de Ítem:
articleDirección web:
https://www.ijimai.org/journal/bibcite/reference/3106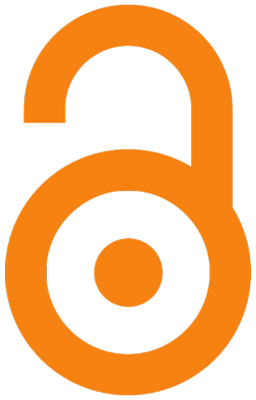
Resumen:
In finance and management, insurance is a product that tends to reduce or eliminate in totality or partially the loss caused due to different risks. Various factors affect house insurance claims, some of which contribute to formulating insurance policies including specific features that the house has. Machine Learning (ML) when brought into the field of insurance would enable seamless formulation of insurance policies with a better performance which will also save time. Various classification algorithms have been used since they have a long history and have also got some modifications for optimum functionality. To illustrate the performance of each of the ML algorithms that we used here, we analyzed an insurance dataset drawn from Zindi Africa competition which is said to be from Olusola Insurance Company in Lagos Nigeria. This study therefore, compares the performance of Logistic Regression (LR), Decision Tree (DT), K-Nearest Neighbor (KNN), Kernel Support Vector Machine (kSVM), Naïve Bayes (NB), and Random Forest (RF) Regressors on a dataset got from Zindi.africa competition and their performances are checked using not only accuracy and precision metrics but also recall, and F1 score metrics, all displayed on the confusion matrix. The accuracy result shows that logistic regression and Kernel SVM both gave 78% but kSVM outperformed LR in precision with a percentage of 70.8% for kSVM and 64.8% for LR showing that kSVM offered the best result.
Ficheros en el ítem
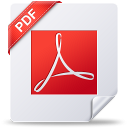
Este ítem aparece en la(s) siguiente(s) colección(es)
Estadísticas de uso
Año |
2012 |
2013 |
2014 |
2015 |
2016 |
2017 |
2018 |
2019 |
2020 |
2021 |
2022 |
2023 |
2024 |
Vistas |
0 |
0 |
0 |
0 |
0 |
0 |
0 |
0 |
0 |
0 |
136 |
584 |
534 |
Descargas |
0 |
0 |
0 |
0 |
0 |
0 |
0 |
0 |
0 |
0 |
150 |
707 |
341 |
Ítems relacionados
Mostrando ítems relacionados por Título, autor o materia.
-
Synthetic Aperture Radar Automatic Target Recognition Based on a Simple Attention Mechanism
Ukwuoma, Chiagoziem Chima; Zhiguang, Qin; Tienin, Bole W.; Yussif, Sophyani B.; Ejiyi, Chukwuebuka Joseph; Urama, Gilbert C.; Ukwuoma, Chibueze D.; Chikwendu, Ijeoma Amuche (International Journal of Interactive Multimedia and Artificial Intelligence (IJIMAI), 12/2023)A simple but effective channel attention module is proposed for Synthetic Aperture Radar (SAR) Automatic Target Recognition (ATR). The channel attention technique has shown recent success in improving Deep Convolutional ... -
A deep learning architecture for power management in smart cities
Xin, Qin; Alazab, Mamoun; García Díaz, Vicente; Montenegro-Marin, Carlos Enrique; González-Crespo, Rubén (Elsevier Ltd, 2022)Sustainable energy management is an inexpensive approach for improved energy use. However, the research used does not focus on cutting-edge technology possibilities in an Internet of things (IoT). This paper includes the ... -
AI-based quality of service optimization for multimedia transmission on Internet of Vehicles (IoV) systems
Xin, Qin; Alazab, Mamoun; González-Crespo, Rubén ; Montenegro-Marin, Carlos Enrique (Sustainable Energy Technologies and Assessments, 2022)Multimedia Communications of Internet of Vehicles (IoV) uses WLAN, NFC and Fifth Generation networks. At the same time, in multimedia communications in healthcare, IoV's essential task is optimizing the quality of experience ...