An Empiric Analysis of Wavelet-Based Feature Extraction on Deep Learning and Machine Learning Algorithms for Arrhythmia Classification
Autor:
Singh, Ritu
; Rajpal, Navin
; Mehta, Rajesh
Fecha:
06/2021Palabra clave:
Revista / editorial:
International Journal of Interactive Multimedia and Artificial Intelligence (IJIMAI)Tipo de Ítem:
articleDirección web:
https://www.ijimai.org/journal/bibcite/reference/2836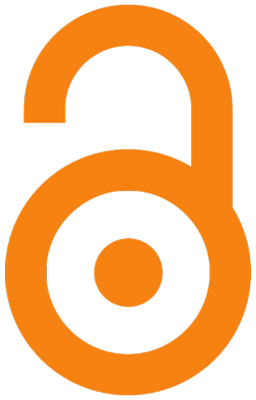
Resumen:
The aberration in human electrocardiogram (ECG) affects cardiovascular events that may lead to arrhythmias. Many automation systems for ECG classification exist, but the ambiguity to wisely employ the in-built feature extraction or expert based manual feature extraction before classification still needs recognition. The proposed work compares and presents the enactment of using machine learning and deep learning classification on time series sequences. The two classifiers, namely the Support Vector Machine (SVM) and the Bi-directional Long Short-Term Memory (BiLSTM) network, are separately trained by direct ECG samples and extracted feature vectors using multiresolution analysis of Maximal Overlap Discrete Wavelet Transform (MODWT). Single beat segmentation with R-peaks and QRS detection is also involved with 6 morphological and 12 statistical feature extraction. The two benchmark datasets, multi-class, and binary class, are acquired from the PhysioNet database. For the binary dataset, BiLSTM with direct samples and with feature extraction gives 58.1% and 80.7% testing accuracy, respectively, whereas SVM outperforms with 99.88% accuracy. For the multi-class dataset, BiLSTM classification accuracy with the direct sample and the extracted feature is 49.6% and 95.4%, whereas SVM shows 99.44%. The efficient statistical workout depicts that the extracted feature-based selection of data can deliver distinguished outcomes compared with raw ECG data or in-built automatic feature extraction. The machine learning classifiers like SVM with knowledge-based feature extraction can equally or better perform than Bi-LSTM network for certain datasets.
Ficheros en el ítem
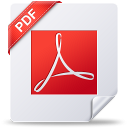
Este ítem aparece en la(s) siguiente(s) colección(es)
Estadísticas de uso
Año |
2012 |
2013 |
2014 |
2015 |
2016 |
2017 |
2018 |
2019 |
2020 |
2021 |
2022 |
2023 |
2024 |
2025 |
Vistas |
0 |
0 |
0 |
0 |
0 |
0 |
0 |
0 |
0 |
0 |
104 |
160 |
201 |
1 |
Descargas |
0 |
0 |
0 |
0 |
0 |
0 |
0 |
0 |
0 |
0 |
57 |
66 |
91 |
0 |
Ítems relacionados
Mostrando ítems relacionados por Título, autor o materia.
-
Predictive text analysis using eye blinks
Chaudhary, Gopal; Lamba, Puneet Singh; Jolly, Harman Singh; Poply, Sakaar; Khari, Manju; Verdú, Elena (Elsevier Ltd, 2021)The current work aims to facilitate interaction with others to those with the inability to perform activities requiring motor skills or those who cannot speak. It proposes a modus operandi or a system based on Histogram ... -
Infected Fruit Part Detection using K-Means Clustering Segmentation Technique
Dubey, Shiv Ram; Dixit, Pushkar; Singh, Nishant; Gupta, Jay Prakash (International Journal of Interactive Multimedia and Artificial Intelligence (IJIMAI), 06/2013)Nowadays, overseas commerce has increased drastically in many countries. Plenty fruits are imported from the other nations such as oranges, apples etc. Manual identification of defected fruit is very time consuming. This ... -
Robust Lossless Semi Fragile Information Protection in Images
Dixit, Pushkar; Singh, Nishant; Prakash Gupta, Jay (International Journal of Interactive Multimedia and Artificial Intelligence (IJIMAI), 06/2014)Internet security finds it difficult to keep the information secure and to maintain the integrity of the data. Sending messages over the internet secretly is one of the major tasks as it is widely used for passing the ...