Semi-Supervised Machine Learning Approaches for Thyroid Disease Prediction and its Integration With the Internet of Everything
Autor:
Agraz, Melih
Fecha:
07/2024Palabra clave:
Revista / editorial:
International Journal of Interactive Multimedia and Artificial Intelligence (IJIMAI)Citación:
M. Agraz. Semi-Supervised Machine Learning Approaches for Thyroid Disease Prediction and its Integration With the Internet of Everything, International Journal of Interactive Multimedia and Artificial Intelligence, (2024), http://dx.doi.org/10.9781/ijimai.2024.07.006Tipo de Ítem:
article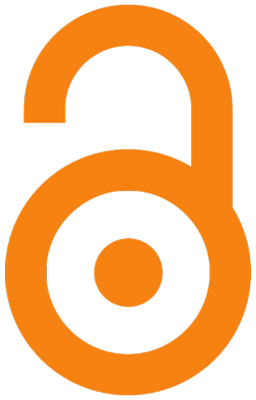
Resumen:
Thyroid disorders are critical conditions that considerably affect a person’s general health, and may lead to additional health complications. Notably, these conditions often remain undetected in individuals who show "normal" results on traditional thyroid function tests. To enhance the diagnostic accuracy for thyroid disorders, such as hypothyroidism and hyperthyroidism, this study leveraged digital health records and explored semisupervised learning methods. We intentionally removed the labels from subjects initially categorized as "normal," incorporating them into our dataset as unlabeled data. The goal was to overcome the limitations of conventional diagnostic techniques, which may fail to detect subtle imbalances in thyroid hormones. In pursuit of this objective, we employed a combination of semi-supervised learning methods, namely FixMatch, Co-training, and self-training, in conjunction with supervised learning algorithms, specifically Naive Bayes and logistic regression. Our findings indicate that the FixMatch algorithm surpassed traditional supervised learning methods in various metrics, including accuracy (0.9054), sensitivity (0.9494), negative predictive value (0.9365), and F1 score (0.9146). Additionally, we propose a framework for integrating these diagnostic tools into the Internet of Everything (IoE) to promote early detection and facilitate improved healthcare outcomes. This research highlights the potential of semi-supervised learning techniques in the diagnosis of thyroid disorders and offers a roadmap for harnessing the IoE in healthcare advancement.
Ficheros en el ítem
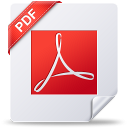
Nombre: Semi-Supervised Machine Learning Approaches.pdf
Tamaño: 1.350Mb
Formato: application/pdf
15 readers on Mendeley
Este ítem aparece en la(s) siguiente(s) colección(es)
Estadísticas de uso
Año |
2012 |
2013 |
2014 |
2015 |
2016 |
2017 |
2018 |
2019 |
2020 |
2021 |
2022 |
2023 |
2024 |
2025 |
Vistas |
0 |
0 |
0 |
0 |
0 |
0 |
0 |
0 |
0 |
0 |
0 |
0 |
153 |
43 |
196 |
Descargas |
0 |
0 |
0 |
0 |
0 |
0 |
0 |
0 |
0 |
0 |
0 |
0 |
70 |
65 |
135 |