A Comparative Analysis of Machine Learning Models for Banking News Extraction by Multiclass Classification With Imbalanced Datasets of Financial News: Challenges and Solutions
Autor:
Dogra, Varun
; Verma, Sahil
; Verma, Kavita
; Jhanjhi, NZ
; Ghosh, Uttam
; Le, Dac-Nhuong
Fecha:
03/2022Palabra clave:
Revista / editorial:
International Journal of Interactive Multimedia and Artificial Intelligence (IJIMAI)Tipo de Ítem:
articleDirección web:
https://www.ijimai.org/journal/bibcite/reference/3100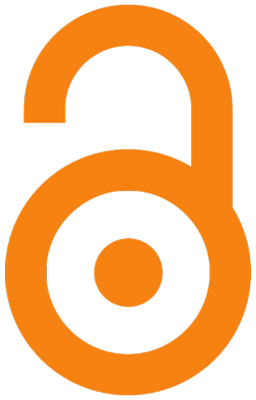
Resumen:
Online portals provide an enormous amount of news articles every day. Over the years, numerous studies have concluded that news events have a significant impact on forecasting and interpreting the movement of stock prices. The creation of a framework for storing news-articles and collecting information for specific domains is an important and untested problem for the Indian stock market. When online news portals produce financial news articles about many subjects simultaneously, finding news articles that are important to the specific domain is nontrivial. A critical component of the aforementioned system should, therefore, include one module for extracting and storing news articles, and another module for classifying these text documents into a specific domain(s). In the current study, we have performed extensive experiments to classify the financial news articles into the predefined four classes Banking, Non-Banking, Governmental, and Global. The idea of multi-class classification was to extract the Banking news and its most correlated news articles from the pool of financial news articles scraped from various web news portals. The news articles divided into the mentioned classes were imbalanced. Imbalance data is a big difficulty with most classifier learning algorithms. However, as recent works suggest, class imbalances are not in themselves a problem, and degradation in performance is often correlated with certain variables relevant to data distribution, such as the existence in noisy and ambiguous instances in the adjacent class boundaries. A variety of solutions to addressing data imbalances have been proposed recently, over-sampling, down-sampling, and ensemble approach. We have presented the various challenges that occur with data imbalances in multiclass classification and solutions in dealing with these challenges. The paper has also shown a comparison of the performances of various machine learning models with imbalanced data and data balances using sampling and ensemble techniques. From the result, it’s clear that the performance of Random Forest classifier with data balances using the over-sampling technique SMOTE is best in terms of precision, recall, F-1, and accuracy. From the ensemble classifiers, the Balanced Bagging classifier has shown similar results as of the Random Forest classifier with SMOTE. Random forest classifier's accuracy, however, was 100% and it was 99% with the Balanced Bagging classifier.
Ficheros en el ítem
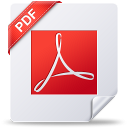
Este ítem aparece en la(s) siguiente(s) colección(es)
Estadísticas de uso
Año |
2012 |
2013 |
2014 |
2015 |
2016 |
2017 |
2018 |
2019 |
2020 |
2021 |
2022 |
2023 |
2024 |
Vistas |
0 |
0 |
0 |
0 |
0 |
0 |
0 |
0 |
0 |
0 |
154 |
572 |
466 |
Descargas |
0 |
0 |
0 |
0 |
0 |
0 |
0 |
0 |
0 |
0 |
106 |
236 |
212 |
Ítems relacionados
Mostrando ítems relacionados por Título, autor o materia.
-
Deep Multi-Model Fusion for Human Activity Recognition Using Evolutionary Algorithms
Verma, Kamal Kant; Singh, Brij Mohan (International Journal of Interactive Multimedia and Artificial Intelligence (IJIMAI), 12/2021)Machine recognition of the human activities is an active research area in computer vision. In previous study, either one or two types of modalities have been used to handle this task. However, the grouping of maximum ... -
Accurate location estimation of moving object In Wireless Sensor network
Bhaskar Semwal, Vijay; Bhaskar Semwal, Vinay; Sati, Meenakshi; Verma, Dr.Shirshu (International Journal of Interactive Multimedia and Artificial Intelligence (IJIMAI), 12/2011)One of the central issues in wirless sensor networks is track the location, of moving object which have overhead of saving data, an accurate estimation of the target location of object with energy constraint .We do not ... -
An analytic Study of the Key Factors Influencing the Design and Routing Techniques of a Wireless Sensor Network
Punetha, Deepak; Bahuguna, Yogita; Verma, Pooja (International Journal of Interactive Multimedia and Artificial Intelligence (IJIMAI), 03/2017)A wireless sensor network contains various nodes having certain sensing, processing & communication capabilities. Actually they are multifunctional battery operated nodes called motes. These motes are small in size & battery ...